Machine Learning Data Labeling: Unlocking the Future of Business Intelligence
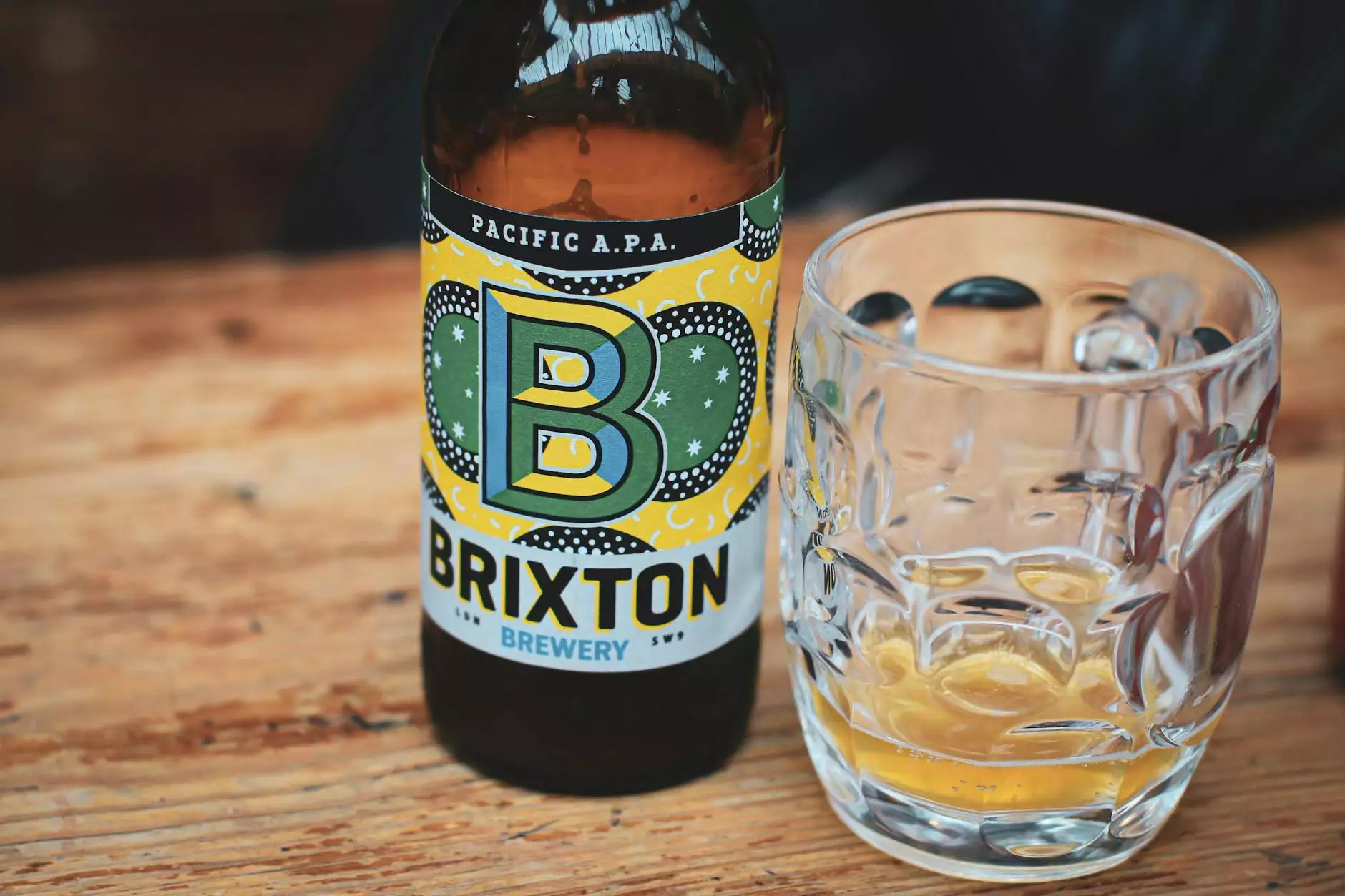
Machine learning data labeling is a crucial process that empowers businesses to harness the full potential of their data. As organizations continue to rely on data-driven decisions, understanding the intricacies of data labeling becomes essential. In this article, we will explore the various aspects of machine learning data labeling, its importance, and how the right tools and platforms can elevate your business capabilities.
The Significance of Data Labeling in Machine Learning
Data labeling involves the process of annotating data—whether it be images, text, or audio—so that machine learning algorithms can interpret and learn from it accurately. This is essential because machine learning models require high-quality, labeled datasets to make predictions or classifications. Here are some key points regarding the significance of data labeling:
- Improves Model Accuracy: Well-labeled data is critical for training accurate models. Accurate labels help algorithms to discern patterns and make better predictions.
- Facilitates Understanding: Labeling provides context to raw data, enabling machines to understand and categorize data like humans do.
- Enhances Learning Efficiency: Labeled data accelerates the training time of models by making it easier for them to learn from examples.
- Reduces Bias: Properly labeled data can minimize biases and improve fairness in AI decision-making processes.
How Machine Learning Data Labeling Benefits Businesses
Businesses across various industries are recognizing the transformative benefits of machine learning data labeling. By effectively labeling data, organizations can:
1. Drive Improved Customer Insights
With data labeling, businesses can analyze customer behavior and preferences more accurately. For instance, sentiment analysis on customer feedback can provide insights into customer satisfaction and areas for improvement.
2. Optimize Operational Efficiency
Data labeling aids in automating routine tasks by training models to recognize patterns. For example, in manufacturing, labeled data can be used to train systems to detect defects in products, streamlining quality control processes.
3. Enhance Marketing Strategies
By analyzing labeled customer data, businesses can personalize marketing efforts. Targeted advertising based on labeled consumer behavior leads to higher conversion rates.
4. Support Predictive Analytics
Labeled historical data assists businesses in predicting future trends. This predictive capability can significantly impact inventory management, demand forecasting, and financial planning.
Key Components of an Effective Data Annotation Tool
Selecting the right data annotation tool is vital for successful machine learning data labeling. Here are the essential features to consider:
- User-Friendly Interface: An intuitive interface simplifies the annotation process, allowing users to focus on data rather than navigating complex software.
- Versatility: The tool should support various types of data, such as images, text, videos, and audio files, to cater to diverse business needs.
- Collaboration Features: Look for tools that allow teams to collaborate in real-time, providing feedback and ensuring consistency in data labeling.
- Quality Control Mechanisms: Robust quality assurance features, such as double-checking annotations or expert reviews, ensure the accuracy of labeled data.
- Integration Capabilities: The ability to integrate with other systems and platforms enhances data flow and usability.
Data Annotation Platforms: Your Path to Excellence
Utilizing a comprehensive data annotation platform can significantly ease the burden of data labeling. These platforms offer a suite of tools designed to improve the efficiency and accuracy of data annotation processes. Here’s why investing in a data annotation platform is crucial:
1. Scalability
As your datasets grow, a robust data annotation platform can scale to manage large amounts of data without compromising quality. This means your business can keep pace with increasing data demands.
2. Automation Support
Many platforms incorporate automated labeling features that help expedite the initial labeling process. This automation reduces manual effort, allowing teams to focus on quality control.
3. Expert Human Oversight
While automation is beneficial, human oversight is still essential. A good platform combines machine speed with human expertise to ensure labels are accurate and contextually relevant.
4. Customizable Workflows
Every organization has unique requirements. A premier platform offers customizable workflows that align with specific business processes, ensuring efficiency and coherence in data handling.
Real-world Applications of Machine Learning Data Labeling
The applications of machine learning data labeling are diverse, impacting various sectors profoundly:
1. Healthcare
In the healthcare industry, labeled data is used to train models for diagnosing diseases, predicting patient outcomes, and personalizing treatment plans.
2. Autonomous Vehicles
Self-driving technology relies heavily on labeled images and videos for object detection and scene understanding, making data labeling a foundational aspect of vehicle safety systems.
3. Finance
In finance, data labeling is critical for fraud detection systems. By labeling transactions as fraudulent or legitimate, machine learning models can identify anomalies effectively.
4. Retail
Retailers utilize labeled customer data to enhance inventory management, recommend products, and improve customer experiences through personalized marketing.
Challenges in Machine Learning Data Labeling
While the benefits of machine learning data labeling are substantial, several challenges need to be addressed:
1. Time and Resource Intensive
Data labeling can be a laborious process, often requiring significant time investment, especially for large datasets.
2. Quality Assurance
Ensuring the accuracy and consistency of labeled data can be challenging. Quality control processes are essential to maintain standards.
3. Scalability Issues
As data volume increases, maintaining an effective workflow and ensuring timely delivery of labeled data can become problematic.
KeyLabs.ai: The Premier Choice for Data Annotation Needs
At KeyLabs.ai, we provide top-notch data annotation tools and platforms designed to streamline machine learning data labeling. Our solutions are equipped with advanced features tailored to enhance your business intelligence capabilities. Here’s what sets us apart:
- Expert Annotations: Our team of specialists ensures high-quality labeling to maximize model performance.
- Advanced Technology: We leverage cutting-edge technology to facilitate rapid and accurate data annotation.
- Your Data, Your Way: Our customizable solutions adapt to your unique business needs, ensuring you get the most out of your data.
- Ongoing Support: Our dedicated support team is always available to assist you throughout the annotation process.
Conclusion
In conclusion, machine learning data labeling is an indispensable component of modern business strategy. As businesses strive to leverage their data effectively, understanding and implementing high-quality data labeling processes will set them apart in a competitive landscape. Embrace the power of precise annotations and unlock the full potential of your data with KeyLabs.ai. By choosing the right tools and platforms, your organization can thrive in the data-driven era.