The Importance of Medical Image Annotation in Healthcare
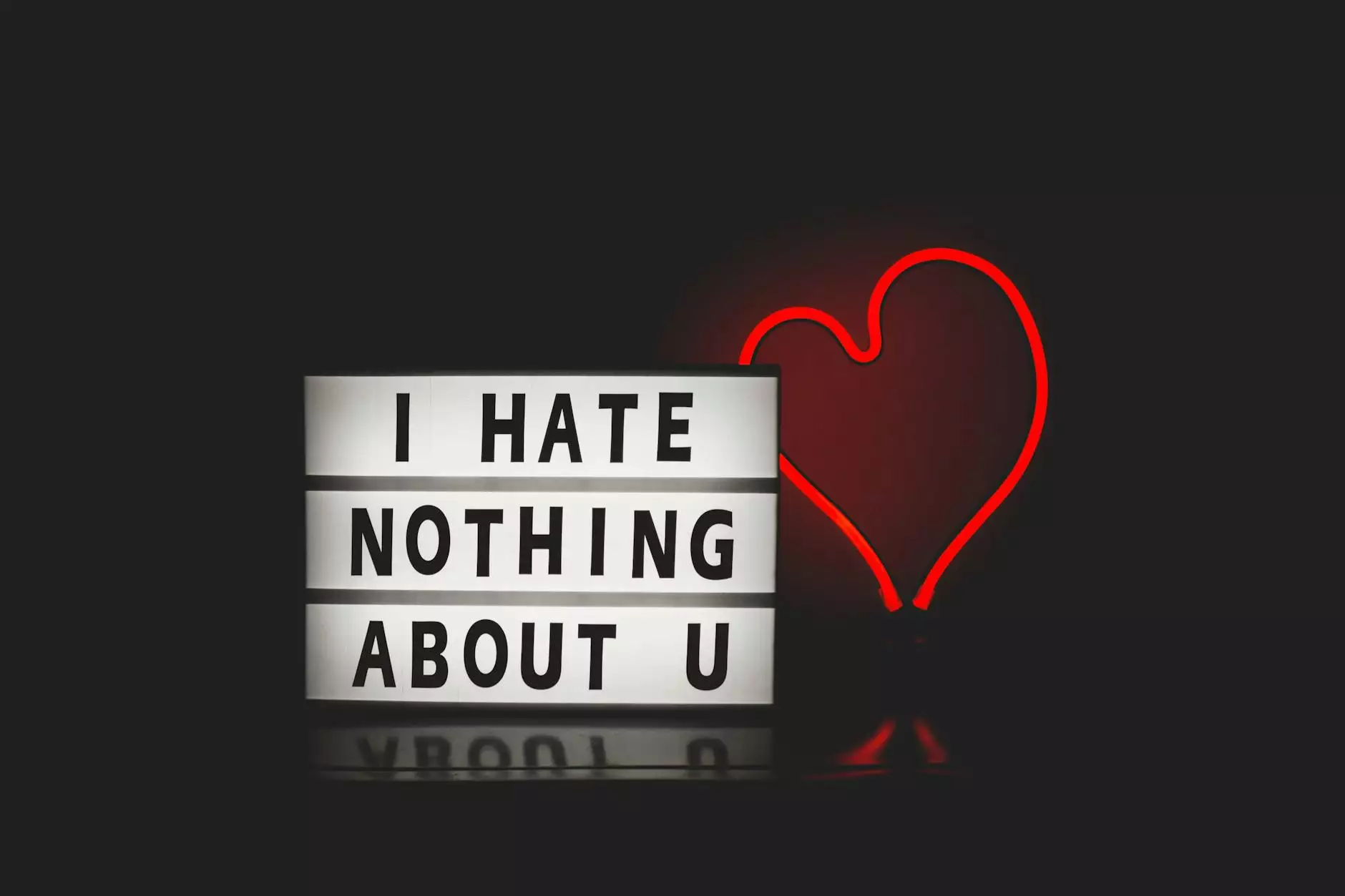
Medical image annotation has become an essential aspect of modern healthcare, facilitating the accurate analysis and interpretation of complex medical data. As healthcare technology evolves, the significance of annotating medical images not only aids in diagnosis but also contributes significantly to the development of advanced machine learning algorithms. In this article, we will delve into the intricacies of medical image annotation, its applications, benefits, and the future it holds in improving patient care and outcomes.
What is Medical Image Annotation?
Medical image annotation refers to the process of labeling or tagging images obtained through various medical imaging techniques, such as X-rays, MRIs, CT scans, and ultrasounds. This process involves identifying and delineating specific structures, lesions, or abnormalities within the images. By providing detailed descriptions and labels, medical image annotation enables healthcare professionals to better analyze and interpret these images, leading to more accurate diagnoses.
Key Techniques in Medical Image Annotation
Several techniques are employed in the process of medical image annotation, each serving different purposes:
- Bounding Box Annotation: This technique involves drawing rectangles around objects of interest in the image, such as tumors or organs, to indicate their location.
- Polygon Annotation: More precise than bounding boxes, this method involves outlining the shape of the objects closely, which is particularly useful for irregularly shaped structures.
- Semantic Segmentation: This process categorizes each pixel in the image and assigns it a label, allowing for a detailed understanding of the various tissues and structures present.
- 3D Annotation: Involves annotating 3D medical models created from imaging data, allowing for a comprehensive analysis of spatial relationships within the anatomy.
The Role of Medical Image Annotation in Diagnosis
The accurate interpretation of medical images is critical in diagnosing diseases. Through medical image annotation, radiologists and clinicians can enhance their analysis, leading to improved detection of conditions such as:
- Cancers: Accurate annotation helps in locating tumors and assessing their size and stage.
- Cardiovascular Diseases: Annotated images enable the assessment of heart conditions by identifying blockages and abnormal structures.
- Neurological Disorders: Brain scans can reveal abnormalities through detailed annotation, aiding in the diagnosis of diseases like Alzheimer's and multiple sclerosis.
- Musculoskeletal Disorders: Annotations can highlight issues in bones and joints, assisting in diagnosing fractures or arthritis.
Advancements in AI and Machine Learning
With the rise of artificial intelligence (AI) and machine learning (ML), medical image annotation has seen a transformative shift. Annotated datasets are crucial for training machine learning models, enabling them to learn and make predictions based on visual data.
AI algorithms can analyze thousands of annotated images to identify patterns that may not be apparent to the human eye. As these algorithms evolve, they can assist healthcare professionals by:
- Enhancing Speed: AI can quickly analyze medical images, drastically reducing the time required for diagnosis.
- Increasing Accuracy: Machine learning models trained on annotated data can significantly reduce the chances of human error.
- Supporting Decision-Making: AI can provide diagnostic suggestions based on annotated datasets, aiding doctors in making informed decisions.
Challenges in Medical Image Annotation
Despite its importance, medical image annotation is not without challenges. Some of these include:
- Subjectivity: The interpretation of medical images can vary among annotators, leading to inconsistencies.
- Time-Consuming Process: Manual annotation is labor-intensive and can take significant time, delaying patient care.
- Need for Expertise: Effective annotation requires specialized knowledge, as incorrect annotations can lead to misdiagnoses.
The Future of Medical Image Annotation
The future of medical image annotation looks promising as technology continues to evolve. Key trends to watch include:
- Automated Annotation Tools: Advancements in AI are leading to the development of automated tools that can perform annotations with minimal human intervention.
- Integration with Electronic Health Records (EHR): Annotated images will increasingly be integrated with EHR systems, streamlining access for healthcare professionals.
- Collaboration Across Disciplines: The combination of radiology, pathology, and AI will foster collaborative approaches to diagnosing diseases.
Conclusion
In conclusion, medical image annotation is a cornerstone of modern healthcare, significantly influencing diagnostic accuracy and patient outcomes. As we venture further into the realm of advanced technologies, the role of annotation will only grow in importance. By enhancing our ability to analyze complex medical images, we can ensure that healthcare professionals are equipped with the best information necessary for decision-making, ultimately improving the quality of care provided to patients worldwide.
Key Takeaways
- Medical image annotation is essential for precise diagnostic processes in healthcare.
- AI and machine learning are transforming the landscape of medical image analysis.
- While challenges exist, the future of medical image annotation promises exciting advancements.