Understanding Quality Image Annotation for Enhanced Business Performance
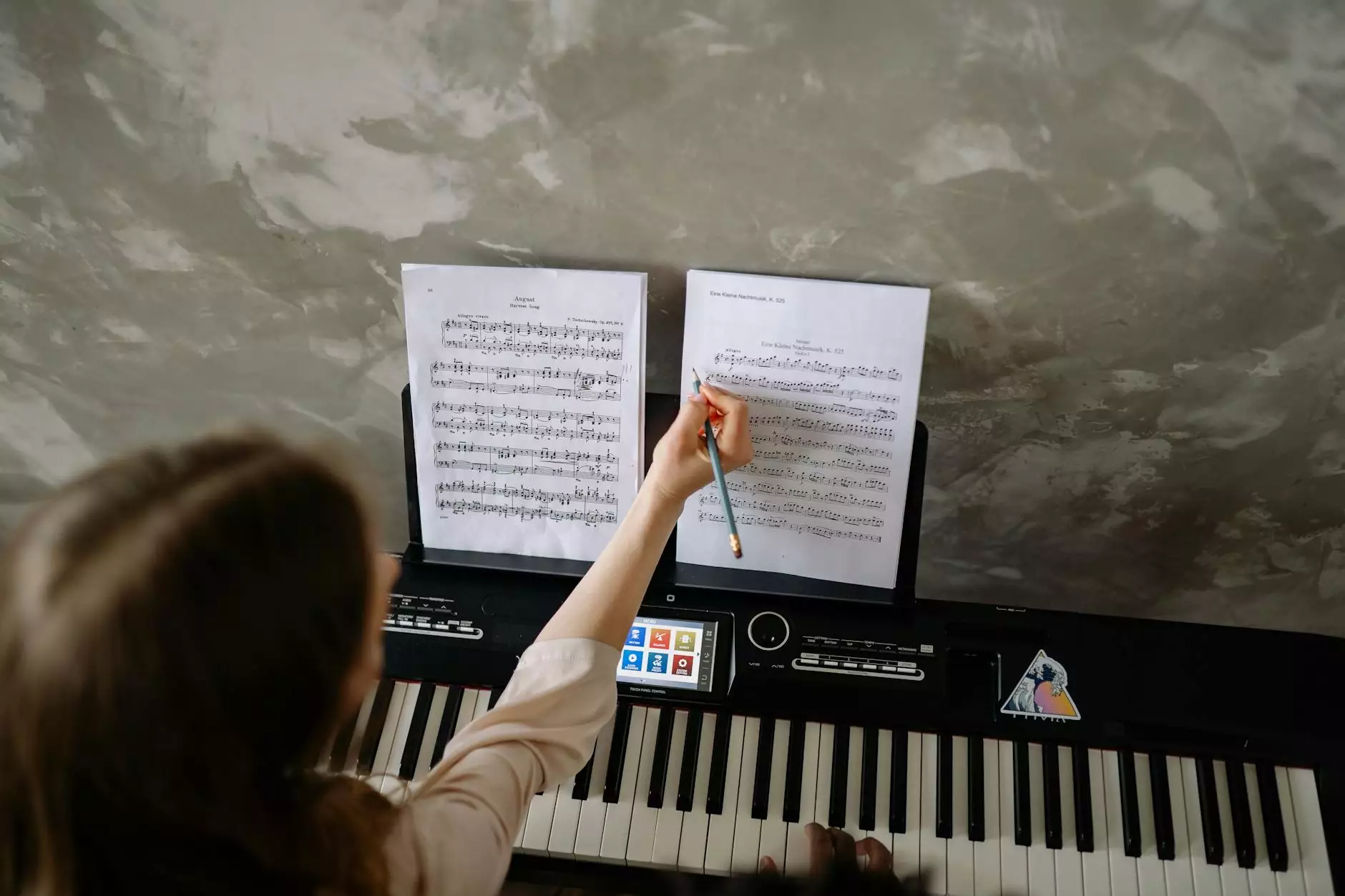
In today's rapidly evolving digital landscape, the significance of quality image annotation cannot be overstated. Businesses, particularly those operating within artificial intelligence (AI) and machine learning (ML) domains, rely heavily on precise and efficient data annotation processes. This article delves deep into the world of image annotation, explaining its importance, the processes involved, and how it can be leveraged to enhance business outcomes.
What is Image Annotation?
Image annotation refers to the process of labeling images with metadata to make them understandable for machine learning algorithms. It lays the groundwork for transforming raw visual data into actionable insights. This process exists at the intersection of data preparation and machine learning, serving as a crucial precursor to any AI project involving computer vision, such as autonomous driving, medical imaging, and facial recognition systems.
The Role of Quality in Image Annotation
When it comes to quality image annotation, precision, and accuracy are paramount. Poor quality annotations can lead to faulty models, resulting in inaccurate predictions and, ultimately, business failure. Here are a few reasons why quality matters:
- Accuracy in Output: High-quality annotations ensure that the models trained on this data perform accurately, thereby increasing reliability.
- Reduction of Errors: Quality assurance in image annotation reduces the chances of errors that can lead to costly consequences.
- Enhanced Learning Algorithms: Well-annotated data helps algorithms learn better, leading to superior performance metrics.
Types of Image Annotation Techniques
Depending on the needs of the business, there are various types of image annotation techniques, each tailored for specific applications:
1. Bounding Box Annotation
This method involves drawing rectangular boxes around objects within an image. It is common in object detection tasks. For example, an image of a cityscape may have boxes around cars, pedestrians, and buildings.
2. Polygon Annotation
Polygon annotation is more precise than bounding boxes, allowing for the mapping of complex shapes. This method is especially useful in medical imaging where lesions may have irregular borders.
3. Semantic Segmentation
This technique involves classifying each pixel in an image to identify the objects present. It is widely used in scenarios like aerial imaging, where land usage classification is necessary.
4. Instance Segmentation
Similar to semantic segmentation but more refined, instance segmentation identifies and segments each occurrence of an object. This is crucial in applications like self-driving cars, where distinguishing between multiple instances is important.
5. Landmark Annotation
This method is used primarily for facial recognition systems, where specific points on a face (like the eyes, nose, and mouth) are annotated to train models on identifying faces in various conditions.
Tools and Platforms for Quality Image Annotation
Businesses today utilize various data annotation tools and platforms to streamline the annotation process:
1. Keylabs.ai
Keylabs.ai is an industry leader in providing advanced image annotation solutions. Their platform is designed to offer high-quality image annotation services, significantly accelerating the development process for AI applications.
2. Labelbox
This platform provides an intuitive user interface for creating, managing, and collaborating on datasets, making it suitable for businesses of all sizes.
3. Supervisely
One of the most robust annotation platforms, Supervisely allows for large-scale projects with extensive collaboration features, making it a great choice for larger businesses.
Benefits of Implementing Quality Image Annotation
Quality image annotation offers numerous benefits that can enhance a business's operational efficiency:
- Improved Model Performance: The better the quality of the annotated data, the more accurate the machine learning models will be.
- Faster Development Cycles: Efficient annotation processes speed up model training phases, allowing businesses to bring products to market faster.
- Cost-Effectiveness: Investing in quality annotation can save businesses from errors that lead to costly corrections downstream.
- Scalability: As businesses grow, having a reliable data annotation strategy in place allows for scalability without compromising quality.
Challenges in Quality Image Annotation
While the benefits are significant, the path to achieving quality image annotation is fraught with challenges:
1. Labor-Intensive Process
Despite advancements in automation, many forms of image annotation still require human oversight, making it labor-intensive and time-consuming.
2. Quality Control
Ensuring high standards of quality across all annotated images can be daunting, especially with large datasets. Implementing consistent quality checks is crucial.
3. Diverse Requirements
Different projects may require unique annotation types, necessitating versatile teams equipped with specialized knowledge.
Best Practices for Achieving Quality Image Annotation
To overcome the challenges and ensure top-tier quality in image annotation, businesses should adopt several best practices:
1. Establish Clear Guidelines
Having well-documented annotation guidelines can significantly improve the consistency and quality of the annotations. These should include clear definitions of objects and proper examples of annotations.
2. Utilize Advanced Annotation Tools
Leveraging cutting-edge data annotation platforms like Keylabs.ai can enhance efficiency and reduce errors.
3. Implement Continuous Training
Regular training sessions for annotators can ensure that they stay updated on best practices and technological advancements in the field.
4. Conduct Regular Quality Audits
Instituting a process for periodic reviews of the annotated data can help identify and rectify quality issues proactively.
Conclusion: The Future of Quality Image Annotation
As businesses increasingly lean into AI and machine learning, the demand for quality image annotation will only grow. The intersection of technology and human expertise is crucial in achieving the desired outcomes from these models. By investing in robust tools, training, and process optimization, companies can position themselves to harness the power of annotated data effectively.
In summary, quality image annotation isn't just a technical requirement; it's a foundational element in driving innovation and success in modern businesses. Companies like Keylabs.ai are at the forefront, facilitating effective transformations that can redefine what's possible in various industries.